July 2024
Boulder, CO
Perennial is excited to announce the publication of our first peer-reviewed scientific paper, "Accurate Quantification of 0–30 cm Soil Organic Carbon in Croplands over the Continental United States Using Machine Learning" in the journal Remote Sensing. This paper represents an important milestone in our mission to unlock agricultural soils as a powerful climate solution.
As one of the first peer-reviewed studies of its kind to be published by a private company, this paper details Perennial’s approach to measuring soil organic carbon (SOC) at depth (below the surface) across US croplands using digital soil mapping. Digital soil mapping uses machine learning models to create detailed maps of soil types and properties by combining information from soil samples with key environmental and biological attributes derived from satellite data. This helps farmers, scientists, and planners understand and manage the land better for agriculture, conservation, and development.
Since conducting this research in 2022, we have made significant headway in further refining our models, reducing the error and uncertainty, and minimizing the density and cost of physical soil sampling.
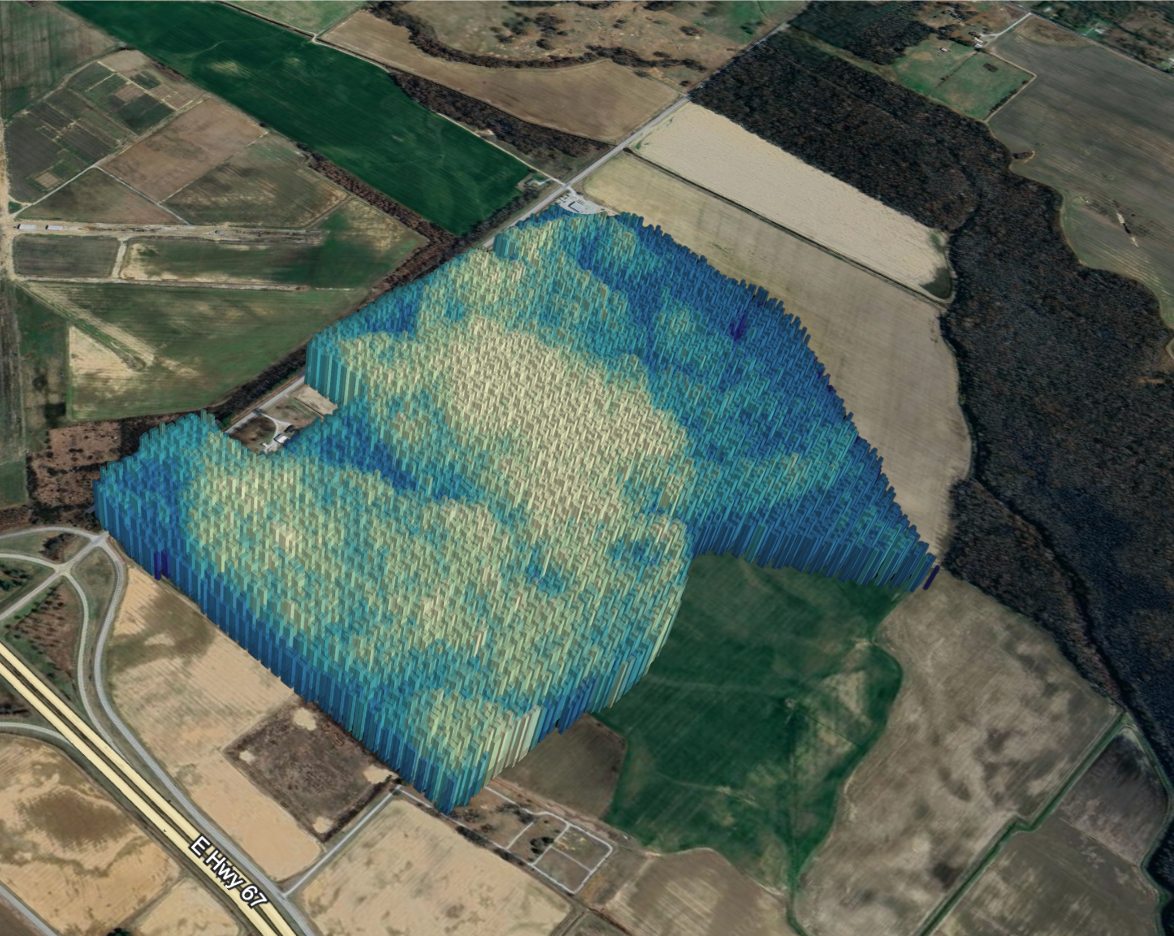
The Importance of Digital Soil Mapping to Specific Depths
Perennial’s research focused specifically on mapping SOC in the 0-30 cm depth range, which is critical for several reasons:
- This upper layer of soil is most responsive to changes in land management practices.
- It aligns with IPCC guidelines for soil carbon accounting.
- It captures a significant portion of the total soil carbon stock in agricultural systems.
Novel Aspects of Perennial’s Research
Our study contributes to the field of DSM by:
- Comprehensive comparison of modeling approaches: We evaluated three machine learning approaches for quantifying SOC at 0-30 cm.
- Integration of diverse data sources: Our models used environmental covariates, including climate data, weather data, topographic information, and remotely sensed data.
- Exploration of legacy data utilization: We investigated how historical soil samples could improve model performance.
- High-resolution output: Our approach enables SOC mapping at a 10-meter resolution across the continental United States.
Key Findings
Our research yielded several important insights:
- Model Performance: Our best-performing model, an XGBoost regression, achieved an R² of 0.48 at the pixel level and 0.64 at the field level for estimating SOC in the 0-30 cm depth range.
- Important Predictors: Remote sensing data and short-term climate/weather variables were the most crucial predictors of SOC content.
- Legacy Data Value: Incorporating legacy soil samples improved model performance.
- Depth Strategy: The full column depth assignment strategy outperformed other depth strategies, and builds on prior studies which show that DSM can accurately model soil carbon content below the soil surface.
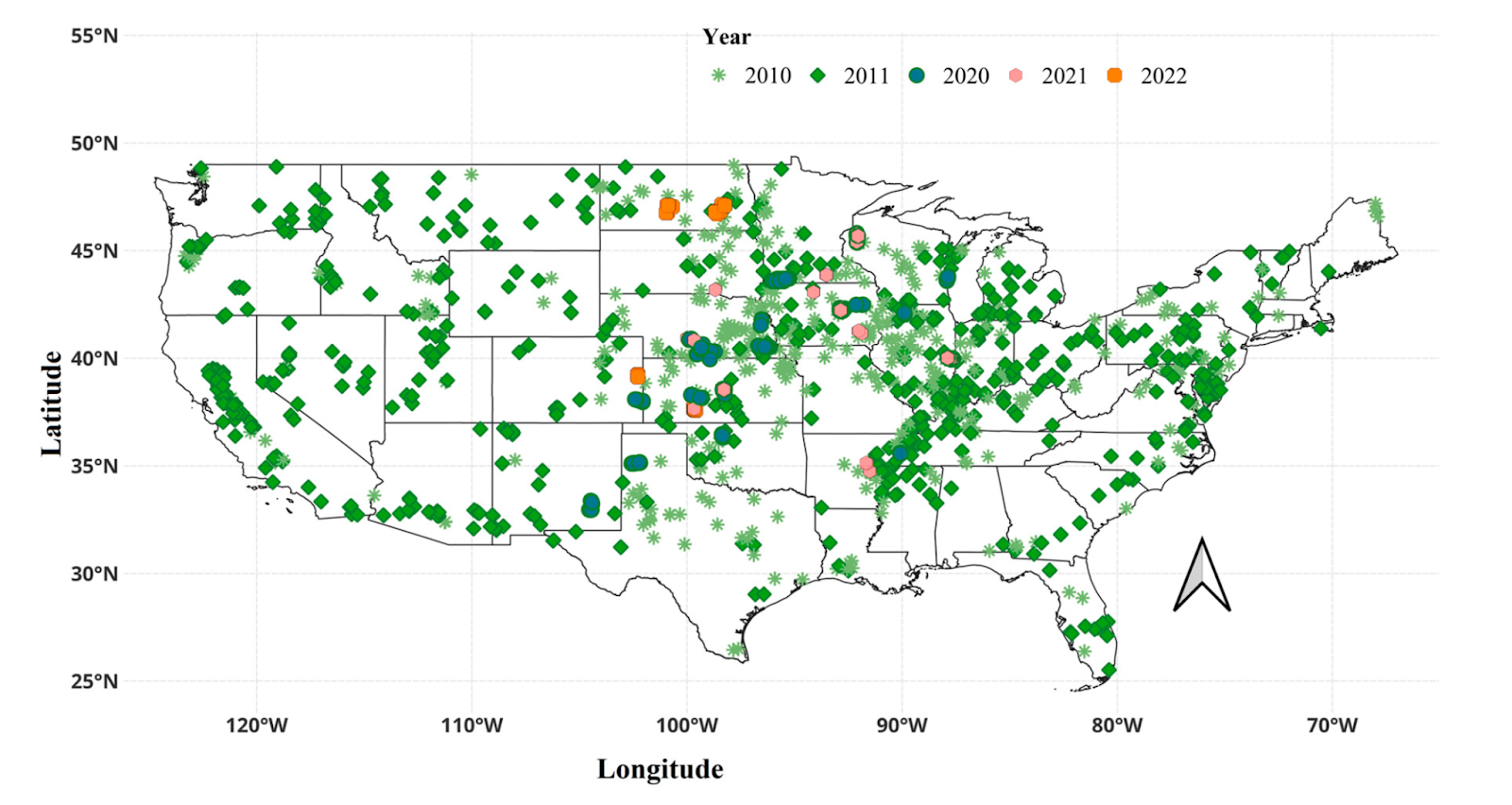
The spatial distribution of soil samples collected from each state across the continental United States (CONUS). The total number of soil samples used in this study was 7018. RaCA soil samples were collected in 2010 and 2011, and the rest of the soil samples were collected between 2020 and 2022.
Implications and Future Decisions
This research lays a strong foundation for scalable, cost-effective soil carbon measurement and monitoring. By reducing reliance on physical soil sampling, Perennial’s approach can significantly lower barriers to participation in carbon markets for farmers and project developers.
At Perennial, we believe that agricultural MRV requires transparency and scientific rigor. We encourage other participants in the sector, particularly other private companies, to pursue peer-review for their work. We will continue to publish our findings and are excited by other research results in our pipeline.
We thank our research collaborators and the peer reviewers for their valuable feedback. The full paper is publicly available [link]. We welcome feedback and discussion from the scientific community as we work to further improve soil carbon quantification at scale.